The Business Intelligence Ecosystem: Understanding the Framework Behind Data-Driven Decisions
In today’s fast-paced business landscape, data is the driving force behind decision-making. The need to process, analyze, and utilize large amounts of information has given rise to the concept of Business Intelligence (BI). At its core, BI encompasses tools, technologies, processes, and practices that turn raw data into actionable insights.
As businesses continue to recognize the value of data, understanding the Business Intelligence ecosystem becomes critical for successful data-driven strategies. This article explores the different components, roles, and segments within the BI ecosystem and how they work together to provide meaningful insights.
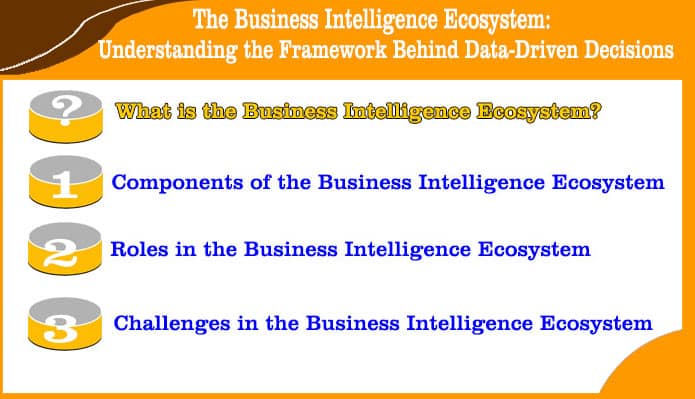
What is the Business Intelligence Ecosystem?
The Business Intelligence ecosystem refers to the interconnected tools, platforms, processes, and practices that enable organizations to collect, store, analyze, and visualize data for strategic decision-making. It includes a range of technologies such as data warehouses, analytics tools, and reporting software, as well as roles like data analysts, IT professionals, and business users.
Unlike a single tool or technology, the BI ecosystem is an entire framework that helps businesses harness the power of their data and transform it into valuable insights.
Components of the Business Intelligence Ecosystem
The BI ecosystem can be broken down into several key components. These components work in tandem to create a seamless process for gathering, analyzing, and presenting data:
1. Data Sources
Data sources form the foundation of the BI ecosystem. These are the systems, databases, and applications where raw data is stored. Data sources can be internal (e.g., customer relationship management (CRM) systems, enterprise resource planning (ERP) platforms, sales databases) or external (e.g., market research reports, social media data, web analytics).
In many organizations, data is stored in multiple locations and formats, which makes data integration and standardization essential before it can be analyzed. Therefore, choosing the right data sources is a critical first step in the BI process.
2. Data Warehousing
Data warehousing plays a pivotal role in the BI ecosystem by centralizing data from different sources into a unified repository. Data warehouses allow businesses to store large amounts of historical data in a structured format, making it easier to perform analysis.
Modern data warehouses support various forms of data—structured, semi-structured, and unstructured—enabling businesses to draw insights from diverse datasets. Additionally, data warehousing tools often include data cleansing and transformation capabilities to ensure data accuracy and consistency.
3. ETL (Extract, Transform, Load) Processes
The Extract, Transform, Load (ETL) process is responsible for transferring data from multiple sources into the data warehouse. During this process, raw data is extracted from its original sources, transformed to ensure it meets the standards required for analysis, and then loaded into the data warehouse for storage.
ETL is a crucial step in the BI ecosystem because it ensures that the data being analyzed is clean, standardized, and ready for reporting. Without efficient ETL processes, businesses risk working with incomplete or inaccurate data, leading to unreliable insights.
4. Data Mining and Analytics
Once data is stored and processed, the next component of the BI ecosystem is data mining and analytics. Data mining involves using algorithms and statistical techniques to discover patterns, correlations, and trends in data. These insights are used for predictive analytics, helping businesses forecast future outcomes and make informed decisions.
Data analytics tools enable businesses to perform deep dives into their datasets, allowing them to uncover hidden opportunities, spot inefficiencies, and optimize performance. There are two primary types of analytics in the BI ecosystem:
- Descriptive Analytics: Focuses on past data to understand historical performance.
- Predictive Analytics: Uses historical data to predict future trends and outcomes.
5. Reporting and Dashboards
Reporting is another essential component of the BI ecosystem, providing users with detailed insights based on their data analysis. BI reporting tools allow users to generate static or dynamic reports, often in the form of spreadsheets, PDFs, or presentations. These reports typically summarize key metrics and performance indicators, helping business leaders understand the current state of their operations.
Dashboards, on the other hand, offer real-time data visualization and present key performance indicators (KPIs) in an easy-to-understand format. Dashboards use graphs, charts, and maps to display data insights visually, enabling users to quickly assess business performance and track progress toward goals.
6. Self-Service BI Tools
Self-service BI tools empower non-technical users to access and analyze data without relying on IT departments or data analysts. These tools provide intuitive interfaces and drag-and-drop functionality, making it easy for business users to create their own reports and dashboards.
The self-service BI segment is gaining popularity as more organizations seek to democratize data access across departments. By enabling employees to generate their own insights, businesses can foster a culture of data-driven decision-making and improve overall efficiency.
7. Cloud-Based BI
The shift toward cloud computing has introduced cloud-based BI as an essential component of the ecosystem. Cloud-based BI platforms offer scalability, flexibility, and cost savings by allowing businesses to store and analyze data in the cloud rather than on-premise servers.
Cloud BI solutions are particularly useful for small and medium-sized businesses that may lack the resources for maintaining complex IT infrastructure. Additionally, cloud BI platforms often offer enhanced collaboration features, enabling teams to share insights and reports in real-time.
8. Artificial Intelligence and Machine Learning
Artificial Intelligence (AI) and Machine Learning (ML) are transforming the BI ecosystem by enabling more advanced data analysis capabilities. AI-powered BI tools can automate data analysis, identify patterns that humans may overlook, and even make recommendations based on predictive models.
Machine Learning algorithms improve over time, making predictions and insights more accurate as new data becomes available. Businesses can use AI and ML in BI for tasks like predictive forecasting, anomaly detection, and personalized recommendations.
Roles in the Business Intelligence Ecosystem
Several key roles play a crucial part in the functioning of the BI ecosystem. Each role has a unique responsibility that contributes to the overall success of BI initiatives:
- Data Analysts: Data analysts are responsible for collecting, processing, and analyzing data. They use various BI tools and techniques to generate insights and create reports that inform business strategies.
- Data Engineers: Data engineers are responsible for building and maintaining the infrastructure that supports the BI ecosystem, such as data warehouses, ETL processes, and data pipelines.
- Business Users: Business users are the end consumers of BI tools and insights. They leverage BI reports and dashboards to make data-driven decisions, improve performance, and achieve business objectives.
- IT Professionals: IT professionals ensure that BI systems and tools are properly implemented, maintained, and secured. They also provide support to users and help with data governance efforts.
Challenges in the Business Intelligence Ecosystem
While the BI ecosystem offers numerous benefits, it also presents challenges:
- Data Quality: Poor data quality can result in inaccurate insights and flawed decision-making. Ensuring that data is clean, consistent, and up-to-date is essential for effective BI.
- Scalability: As businesses grow, so do their data needs. Ensuring that BI tools and infrastructure can scale to accommodate larger datasets is a common challenge.
- User Adoption: Despite the availability of powerful BI tools, getting employees to adopt and use them can be difficult. Organizations must provide adequate training and support to foster a data-driven culture.
Conclusion
The Business Intelligence ecosystem is a complex yet powerful framework that enables organizations to make data-driven decisions. By understanding the key components—data sources, data warehousing, ETL processes, analytics, reporting, and AI—businesses can build robust BI strategies that unlock valuable insights.
As BI continues to evolve, embracing the full ecosystem will allow organizations to stay ahead of the competition, optimize performance, and make smarter, more informed decisions.