The Role of Machine Learning in Business Intelligence: Enhancing Data-Driven Decision Making
As businesses continue to rely on data to drive strategic decisions, Business Intelligence (BI) has emerged as a critical tool for analyzing data and extracting insights. However, with the explosion of data in today’s digital world, traditional BI methods that rely on historical data analysis are no longer sufficient to meet the growing needs of organizations. To overcome these limitations, businesses are turning to machine learning (ML) to enhance BI capabilities.
Machine learning plays a transformative role in Business Intelligence by automating data analysis, uncovering hidden patterns, and enabling predictive and prescriptive analytics. In this article, we will explore the role of machine learning in Business Intelligence, the key benefits it offers, and how it is changing the landscape of data-driven decision-making.
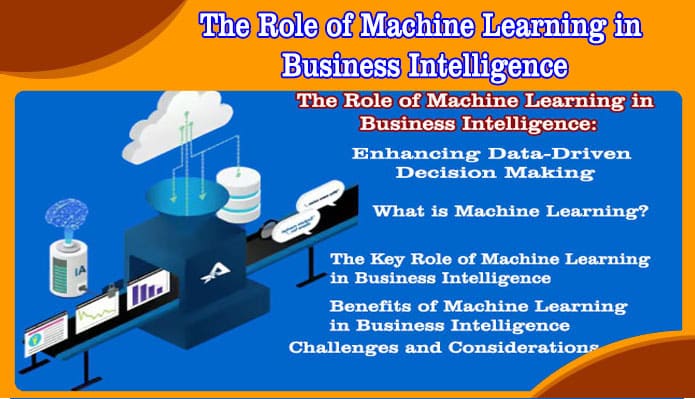
What is Machine Learning?
Machine learning is a branch of artificial intelligence (AI) that allows computers to learn from data and make predictions or decisions without being explicitly programmed. ML algorithms analyze vast datasets, identify patterns, and improve their performance over time as more data becomes available.
In the context of Business Intelligence, machine learning enables organizations to process and analyze massive amounts of data more efficiently and accurately than traditional BI tools. ML can automatically uncover trends, predict future outcomes, and provide actionable insights that help businesses make smarter decisions.
The Key Role of Machine Learning in Business Intelligence
Machine learning plays several important roles in enhancing BI processes. Below are the key areas where ML adds value to BI:
1. Automated Data Processing and Analysis
One of the primary advantages of machine learning in Business Intelligence is its ability to automate data processing and analysis. Traditional BI systems require analysts to manually define queries, clean data, and interpret results. This process can be time-consuming and prone to human error.
Machine learning, however, can automate these tasks, significantly speeding up the data analysis process. By using data preprocessing algorithms, ML can automatically clean and organize data, ensuring that businesses work with high-quality information. This automation not only saves time but also allows analysts to focus on interpreting insights rather than manual data handling.
2. Advanced Data Pattern Recognition
Machine learning is highly effective at recognizing patterns within large datasets that would be difficult or impossible for humans to detect. This is particularly important in industries where understanding complex relationships between variables can drive key business outcomes.
For example, ML algorithms can analyze customer behavior patterns by examining purchase histories, browsing habits, and interactions with marketing campaigns. This can help businesses identify customer segments, personalize marketing efforts, and predict future customer behavior more accurately.
Similarly, in supply chain management, ML can detect patterns related to inventory levels, demand forecasting, and supplier performance, helping businesses optimize operations and reduce costs.
3. Predictive Analytics
Predictive analytics is one of the most significant applications of machine learning in Business Intelligence. Unlike traditional BI systems that focus on historical data, machine learning allows businesses to forecast future trends based on past behavior and current conditions.
Using predictive models, machine learning algorithms can analyze large datasets to identify patterns and predict potential outcomes with high accuracy. For example, ML can forecast future sales trends, customer churn rates, or market demand, allowing businesses to be more proactive in their strategies.
Predictive analytics can also be used in areas such as financial planning, where machine learning can help predict cash flow, revenue growth, and investment returns. This enables businesses to make more informed financial decisions and reduce risks.
4. Prescriptive Analytics
Prescriptive analytics takes predictive analytics one step further by recommending specific actions that businesses should take to achieve desired outcomes. Machine learning can analyze the potential impact of various decisions and provide recommendations based on data-driven insights.
For instance, an ML algorithm might suggest that a retail company increase its inventory of a particular product based on predicted demand, or it may recommend personalized promotions for customers likely to churn. These prescriptive recommendations help businesses take proactive steps to improve their operations and profitability.
5. Real-Time Analytics
With the increasing availability of real-time data, machine learning is playing a critical role in enabling real-time analytics. Traditional BI systems often rely on batch processing, which means data is analyzed in intervals, leading to delays in insights.
Machine learning algorithms, however, can process and analyze data in real time, allowing businesses to make decisions based on the most current information available. This is especially valuable in industries where rapid decision-making is essential, such as finance, e-commerce, and logistics.
For example, a financial institution can use machine learning to detect fraudulent transactions in real time, while an e-commerce company can adjust pricing strategies dynamically based on real-time customer behavior and market conditions.
6. Enhanced Data Security and Fraud Detection
Machine learning is also playing a vital role in improving data security and fraud detection within Business Intelligence systems. By analyzing large volumes of data, ML algorithms can identify unusual patterns and detect potential security threats.
For example, in the financial sector, machine learning can analyze transaction data in real time to detect fraudulent activities, such as unusual spending patterns or unauthorized access attempts. Similarly, ML can be used to monitor network traffic in cybersecurity, helping businesses detect and prevent data breaches.
By leveraging machine learning for security, businesses can protect sensitive data, reduce the risk of financial loss, and ensure compliance with regulatory standards.
7. Natural Language Processing (NLP) in BI
Another important role of machine learning in Business Intelligence is the integration of Natural Language Processing (NLP). NLP allows BI systems to understand and interpret human language, making data analysis more accessible to a wider range of users.
With NLP, users can interact with BI tools using natural language queries rather than complex SQL queries or technical jargon. For example, a user can simply ask, “What were the sales trends last quarter?” and the machine learning-powered BI system will analyze the data and provide a relevant answer.
NLP also enables organizations to analyze unstructured data, such as customer reviews, social media posts, and emails. By analyzing sentiment and feedback, businesses can gain valuable insights into customer preferences, brand perception, and areas for improvement.
Benefits of Machine Learning in Business Intelligence
Integrating machine learning into Business Intelligence systems offers numerous benefits for businesses. Some of the key advantages include:
- Faster Decision-Making: Machine learning automates data analysis, allowing businesses to generate insights and make decisions more quickly. This speed is particularly valuable in fast-moving industries where quick responses to market changes are essential.
- Improved Accuracy: Machine learning algorithms can process vast amounts of data with higher accuracy than human analysts, reducing the risk of errors in data analysis and decision-making.
- Deeper Insights: Machine learning can uncover hidden patterns and relationships within data that may not be immediately apparent, providing businesses with deeper insights that drive more effective strategies.
- Proactive Strategy Development: With predictive and prescriptive analytics, machine learning enables businesses to anticipate future trends and take proactive steps to capitalize on opportunities or mitigate risks.
- Personalization: Machine learning allows businesses to deliver personalized experiences to customers by analyzing their behavior and preferences. This leads to improved customer satisfaction and loyalty.
Challenges and Considerations
While machine learning offers significant benefits for Business Intelligence, there are also challenges that organizations need to consider:
- Data Quality: Machine learning relies heavily on the quality of the data it analyzes. Poor-quality data can lead to inaccurate predictions and insights, so businesses need to invest in robust data governance and data cleansing processes.
- Complexity: Implementing machine learning in BI systems can be complex, requiring skilled data scientists and analysts to develop and maintain ML models. Smaller businesses may face resource constraints when adopting ML-powered BI tools.
- Data Privacy: With machine learning algorithms analyzing large datasets, businesses need to ensure they comply with data privacy regulations and protect sensitive information.
Conclusion
The role of machine learning in Business Intelligence is transforming the way businesses analyze data, extract insights, and make decisions. By automating data analysis, enabling predictive and prescriptive analytics, and providing real-time insights, machine learning empowers organizations to stay ahead in an increasingly competitive landscape.
As machine learning continues to advance, its integration with Business Intelligence will become even more critical for organizations looking to harness the full potential of their data. By embracing ML-powered BI tools, businesses can enhance decision-making, improve operational efficiency, and gain a competitive edge in the market.